
A variety of classical reconstruction techniques exist, such as diffusion-based 7–9 and structure/exemplar-based. This process is called inpainting.īroadly, there are two approaches to inpainting – classical statistical reconstruction 6 and machine learning reconstruction. Alternatively, it is possible to replace these occluded regions with reconstructed microstructure post hoc, thus reducing the cost of characterisation.
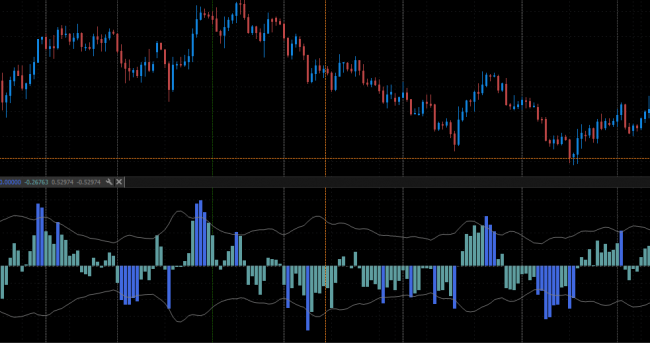
Whilst the imaging would ideally be repeated in the hope of obtaining a clean micrograph (if the imaging technique is not destructive), this is a time consuming process, and particularly challenging if large, representative images are needed.
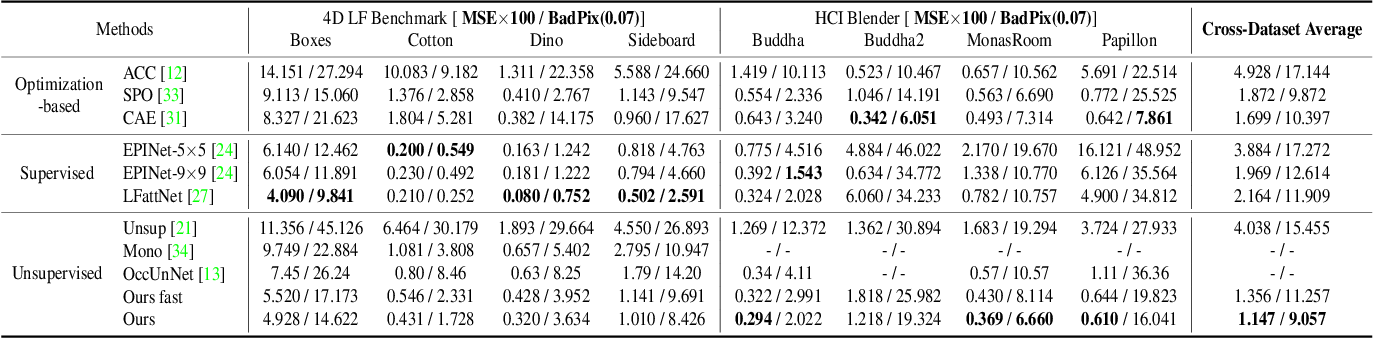
#INPAINT DISPARITY CODE SOFTWARE#
Some common sources of occlusions are surface scratches during sample cutting, 4 charging effects in scanning electron microscopy (SEM), 5 and regions of an image being corrupted by software errors. Unfortunately, samples can suffer from defects during preparation and artefacts can be caused by disturbances during imaging, resulting in regions of the image being unusable for analysis and simulation (hereafter referred to as occluded regions). 1–3 This in turn facilitates material design and optimisation. Microstructural images, alongside statistical image analysis and physical simulations, allow for microstructural features to be linked to material behaviour. 1 Introduction Characterising materials with imaging techniques is critical to understanding their structure–function relationship. We also describe an open-access graphical user interface that allows users to utilise these machine learning methods in a ‘no-code’ environment. We find that one benefits from high speed and simplicity, whilst the other gives smoother boundaries at the inpainting border. In this paper we introduce two methods that use generative adversarial networks to generate contiguous inpainted regions of arbitrary shape and size by learning the microstructural distribution from the unoccluded data. Microstructural inpainting is a method to alleviate this problem by replacing artefacts with synthetic microstructure with matching boundaries. This is particularly problematic for applications where the micrograph is to be used for simulation or feature analysis, as artefacts are likely to lead to inaccurate results. However, even with careful sample preparation and microscope calibration, imaging techniques can contain defects and unwanted artefacts. Imaging is critical to the characterisation of materials.
